AI In Mortgage Origination: Data And Rules
The mortgage industry has long been characterized by manual processes and tedious data entry. However, recent advancements in artificial intelligence (AI) have created tremendous buzz, and lenders of all sizes are taking a deep look at their processes to uncover where human work can be augmented or removed. Given the current economic environment and every lender’s clear focus on finding greater operational efficiency, the world’s “ChatGPT moment” couldn’t have come at a better time for the mortgage industry. AI has the potential to revolutionize mortgage origination and unlock step-change improvements in efficiency and accuracy for lenders — which will not just drive profitability, but improve experiences for borrowers. As mortgage leaders formulate and refine their AI strategy, it’s crucial they understand how these technologies can be applied, pitfalls to avoid, and how they can position themselves to leverage further advancements effectively.
Before we get to the AI: understanding mortgage origination and the problem
Fundamentally, mortgage origination can be boiled down to two things: data and rules. The entire loan manufacturing process is comprised of collecting data — about the borrower, property, and situation — and evaluating that data against a complex set of rules established by regulators and investors. Loans that comply with all applicable rules are considered suitable for origination.
Currently, this process is highly manual due to the unstructured nature of both the data and the rules. Data comes in various forms, including conversations with borrowers (verbal or written), unstructured documents (bank statements, paystubs, etc.), emails from other parties in the transaction (real estate agents, appraisers, etc.), and more. The rules, since usually not driven by individual lenders themselves, are contained within written “guideline” documents that are often 1,000+ pages long. Today, lenders pay humans to “structure” the data — put it into a loan origination system — and then to “run the rules” against the data, which entails reading, understanding, and applying the guidelines to determine loan compliance and saleability. Most technology today is fundamentally not excellent at running unstructured rules against unstructured data, which is where the human cost comes in. The reality is, humans are not excellent at it either, creating a pattern of “checkers checking checkers” and costs that only move in one direction.
Where AI fits in
Rather than trying to use AI to directly solve the problem of “unstructured data + unstructured rules”, a combination of modern AI techniques and existing technology can be employed to translate this problem into a less complex one. In particular, the general intelligence capabilities introduced with the latest foundation models show huge promise in structuring both the data and the rules, thereby converting the issue into a “structured data + structured rules” problem — the exact scenario that existing rules engines are designed to handle. These advanced models can now interpret and “understand” unstructured data, then translate it into a structured format that traditional systems or rules engines can perform evaluation on. Specific use cases include:
Reading documents: Reading and extracting data off of documents, such as bank statements, IDs, paystubs, and more. This is commonly referred to as OCR / ICR (optical / intelligent character recognition).
Audio extraction: Translating call logs into structured text, and automatically inputting relevant data into origination systems.
Codifying rules: Reading and interpreting investor guidelines, and translating them into executable code that (structured) data can be evaluated against.
Regulatory compliance: Monitoring regulatory changes and incorporating updates into structured rules.
These capabilities will eliminate huge amounts of manual data collection and entry, and help automate meaningful portions of the origination process. Once machines can perform these tasks with greater accuracy than humans, efficiency will not only increase, but loan quality and compliance will jump as well — reducing the risk of buybacks.
The power of foundation models
None of the concepts mentioned above are actually particularly novel. In fact, the industry has been working on these solutions for decades, including using AI techniques to translate unstructured data into structured data, read documents, and codify rules. So why is this time different?
The key change lies in a fundamental discovery about artificial intelligence: “bigger is better”. Specifically, investing a billion dollars training a general model creates a super “smart” model that outperforms a thousand smaller, million-dollar models each purpose-built for different tasks. Most importantly, this general model doesn’t just perform better on average – it actually surpasses the small, tailored models at the very tasks they were designed for. The GPT series of models demonstrated to the research community that there are economies of scale in training these models, when they’re large enough, and now the race is on in Silicon Valley.
The mortgage industry can expect to reap huge benefits from these large, “smart” models if we leverage them correctly. No longer do we need to gather 500 samples of each bank statement format to build a specialized model; rather, we can simply query an API provided by the world’s largest tech companies. Trained on trillions of tokens, these models leverage their general understanding of language to extract data from nearly any bank statement, with no additional training.
This simultaneously lowers the barrier to AI adoption — no need to train your own model at all, just get one off the shelf that is very capable of the task you want — and raises it to be insurmountably high — you cannot get a better model than everybody else without spending billions of dollars on NVIDIA chips and AI researchers.
This new dynamic will make powerful AI far more accessible to lenders, enabling anyone to easily structure the data and rules that comprise the mortgage process, and pushing the industry closer to end-to-end automation. What once required years of investment, development, and training has been reduced to a simple API call, with these capabilities continuing to advance.
How lenders should approach implementing AI
While there’s massive potential for AI in mortgage origination, and it will only get better, lenders need to approach its implementation thoughtfully. I suggest a few key considerations:
Understand the business problem: Before implementing any AI solution, clearly define the specific challenges you’re trying to address in your origination process. You should implement AI to solve a problem, not just for the sake of implementing AI.
Leverage existing models: Rather than training custom models, figure out how you can use existing foundation models to solve your problems. These models offer powerful, generalizable capabilities for cheap; try not to build your own solution just to solve a narrow part of your process. We often see procurement and privacy pose questions and challenges here; these are hard and valid objections, but need to be confronted head-on. Trying to invest in AI in 2024 because it’s “hot” without actually leveraging a big foundation model is like trying to run a marathon in old leather sandals – you’re participating in the race, but ignoring advancements in the field and giving yourself an unnecessary handicap.
Patience and persistence: If current AI models aren’t meeting your needs, wait 6-12 months and try again. Large language models have thus far gotten meaningfully better every year. When OpenAI, Microsoft, Google, and Meta are spending billions of dollars a month to make the next model better, it doesn’t make sense for individual lenders to build their own; the base models will catch up in a more generalizable and powerful sense.
Flexible architecture: Nobody can predict the winners of the AI race. As such, the best thing you can do is implement a flexible, open technology architecture so that you can plug in whichever companies or technologies emerge as the most effective for your use cases.
Notable barriers to adoption
Talking about AI is one thing, but actually using it is another. It’s crucial to acknowledge the challenges that come with implementing AI in origination processes, with two key obstacles being data privacy and operational change.
Data privacy poses a significant challenge to lenders because it’s impossible to disentangle the task of “structuring data” from the sensitive information inherent to our industry. For example, for a foundation model to complete a task such as extract text from a borrower-provided document, it must process the personally identifiable information (PII) contained within the document. This presents concerns for most financial institutions, which must adhere to strict information security requirements. Although major providers are investing heavily in making AI solutions more enterprise-ready, particularly in terms of security and privacy, a lot of uncertainty remains.
When it comes to operations, AI faces many of the same challenges that hinder other technologies in our industry — integrations with legacy systems, entrenched processes, and users reluctant to change the way they work. Regardless of the technology being used, there is a huge amount of friction involved in actually cutting costs or removing steps from the mortgage process, and AI is no different. To truly realize significant efficiency gains from AI, meaningful process reinvention is necessary, and that requires buy-in at all levels of both the technology and operations sides of an organization.
The AI-driven future of mortgage origination
AI has tremendous potential to greatly streamline the mortgage origination process. By harnessing AI to structure data, interpret rules, and automate complex tasks, lenders can achieve significant gains in operational efficiency, accuracy, and compliance. While challenges remain, the potential benefits of AI in mortgage origination are too substantial to overlook. Lenders who approach AI implementation with thoughtfulness and patience, prioritize leveraging existing models, and maintain a flexible technology architecture will be well-positioned to lead the next era of mortgage innovation. As AI permeates the mortgage industry, it will not only benefit lenders but also create a more seamless and accessible experience for borrowers. The future of mortgage lending is inextricably linked with AI, and those who embrace this technology wisely will be at the forefront of a transformed industry.
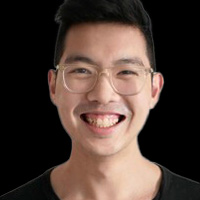
As CEO at Vesta, Mike Yu leads sales, product development, and implementations as the team redefines origination platforms for modern lenders. Previously, Mike spent 4 years on the early product team at Blend, where he launched key components of the flagship mortgage platform, and later started and ran new business lines such as Blend Insurance. Mike graduated from Stanford University with an MS in Computer Science/AI and a BA in Economics.